Unleashing the Potential of Fintech Machine Learning
Machine learning (ML) is a type of artificial intelligence (AI) that allows computer systems to learn and make predictions without being explicitly programmed.Machine learning has had a profound impact on the financial industry, leading to a significant evolution in the way fintech firms operate.
Machine learning (ML) is a type of artificial intelligence (AI) that allows computer systems to learn and make predictions without being explicitly programmed. It involves training algorithms on large datasets and then using the learned patterns to make predictions on new, unseen data.
Here’s a simplified explanation of how machine learning works:
- Data Collection and Preprocessing: the first step in the machine learning process is to collect and prepare the data. This involves cleaning, transforming, and normalizing the data to make it ready for analysis. This is an important step because machine learning algorithms can only learn from well-formatted components.
- Model Selection: select the right type of ML algorithm. There are many solutions available for different use cases. Some of the common algorithms include linear regression, decision trees, random forests, support vector machines and neural networks. The choice depends on the nature of the problem and the type of data.
- Training: once the data is ready and the model is selected, it's time is to train. The algorithm uses the data to learn the patterns and relationships between the features. This is done by adjusting the weights and biases of the model to minimize the error between the predicted and actual outputs.
- Validation: validate the model using a test dataset. This dataset is not used during training, but is used to assess the accuracy of the model. The goal is to find the model that generalizes well to unseen data.
- Deployment: when the model is validated, it is ready to be deployed into a real-world application. This involves integrating the model into the software system, and using it to make predictions on new data.
- Monitoring and Maintenance: the final step is to monitor the performance of the model and make any necessary updates or improvements. This may request retraining the model with new data, adjusting the parameters or even selecting a new algorithm.
Exploring the Applications of Machine Learning in FinTech companies
Machine learning has had a profound impact on the financial industry, leading to a significant evolution in the way fintech firms operate. Here is a closer look at some of the most impactful applications of machine learning in this industry:
Fraud Detection
Machine learning algorithms are extensively utilized in detecting fraud by examining large volumes of transactional data to detect patterns and anomalies that could signal malicious activity. These algorithms can be trained to recognize the typical behavior patterns of customers, allowing them to flag transactions that diverge from the norm.
Risk Management
Financial institutions can benefit greatly from machine learning algorithms in managing risk. The algorithms can provide real-time insights into potential risks, helping institutions identify market trends, predict stock prices, and make informed investment decisions based on thorough data analysis.
Credit Scoring
These solutions have the capability to automate the credit scoring process, by analyzing extensive amounts of data on the creditworthiness of both individuals and businesses. The algorithms can utilize factors such as income, employment status, and payment history to predict the likelihood of default.
Customer Support
The fintech sector can harness the power of ML algorithms to provide personalized and automated customer service. Chatbots, for instance, can be trained to handle common customer queries, freeing up staff to attend to more complex issues.
Portfolio Optimization
AI technology platforms can optimize portfolios by identifying the most profitable investments based on market trends and historical data analysis. They can also create customized portfolios that cater to individual investment goals and risk tolerance levels.
Algorithmic Trading
Algorithmic trading is going to foster investment decisions based on data analysis. These systems can analyze market trends and make predictions on stock prices in real time, allowing traders to make quick and informed investment decisions.
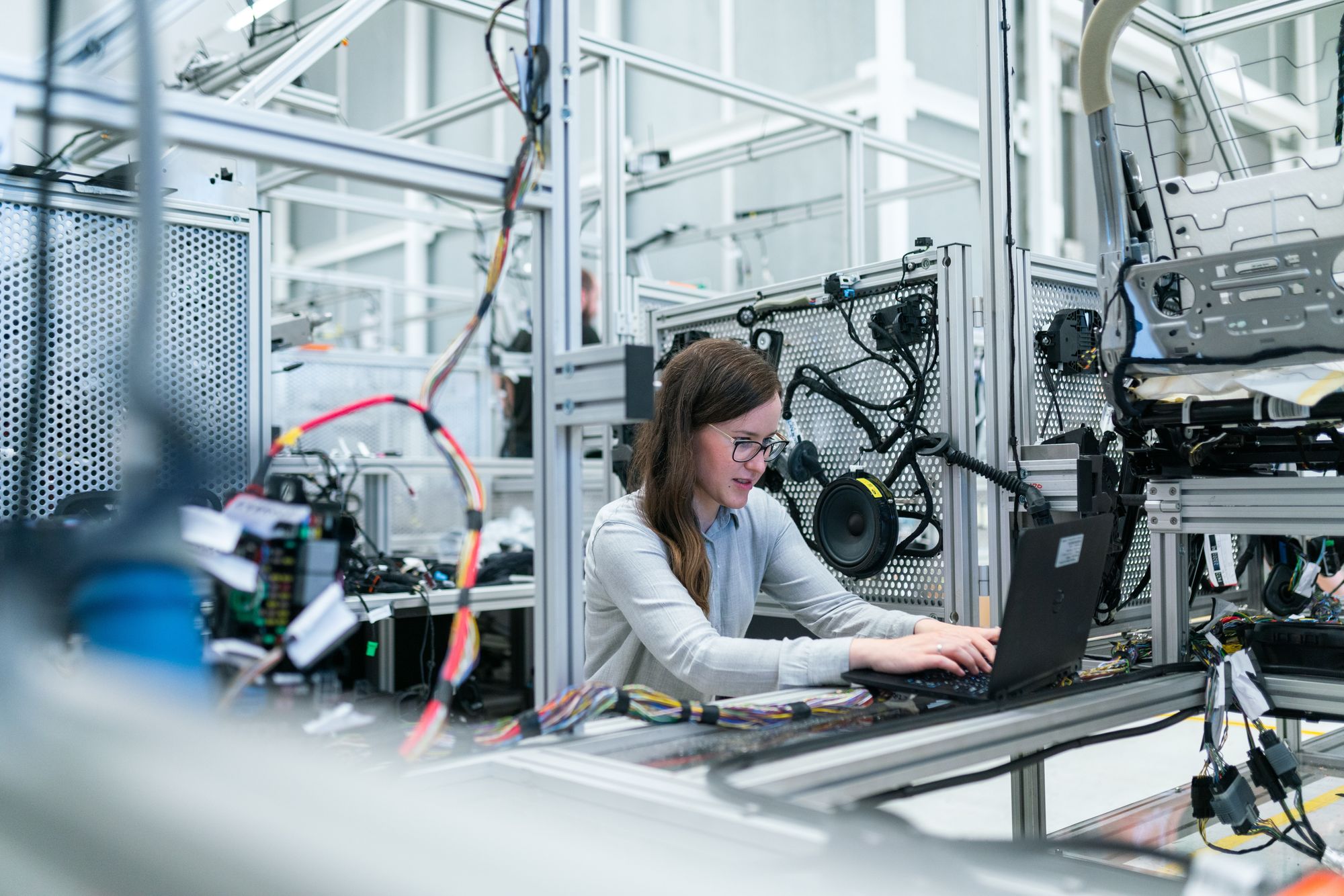
Based on extensive research and analysis, it is clear that machine learning will continue to have a significant impact on this sector in the future. The technology has already revolutionized the way financial institutions operate, improving processes and efficiency in different areas.
The future impact of machine learning on finance is expected to be even more profound, with experts predicting that the technology will continue to transform the industry in new and innovative ways. For example, ML applications are expected to play a major role in the development of next generation financial products and services, such as personalized investment portfolios, automated financial advice and real-time risk assessments.
The potential for machine learning to improve those services is tremendous, and financial institutions that embrace the technology will be well positioned to remain competitive in the years to come. This makes it a critical area for investment for institutions looking to stay ahead of the curve.